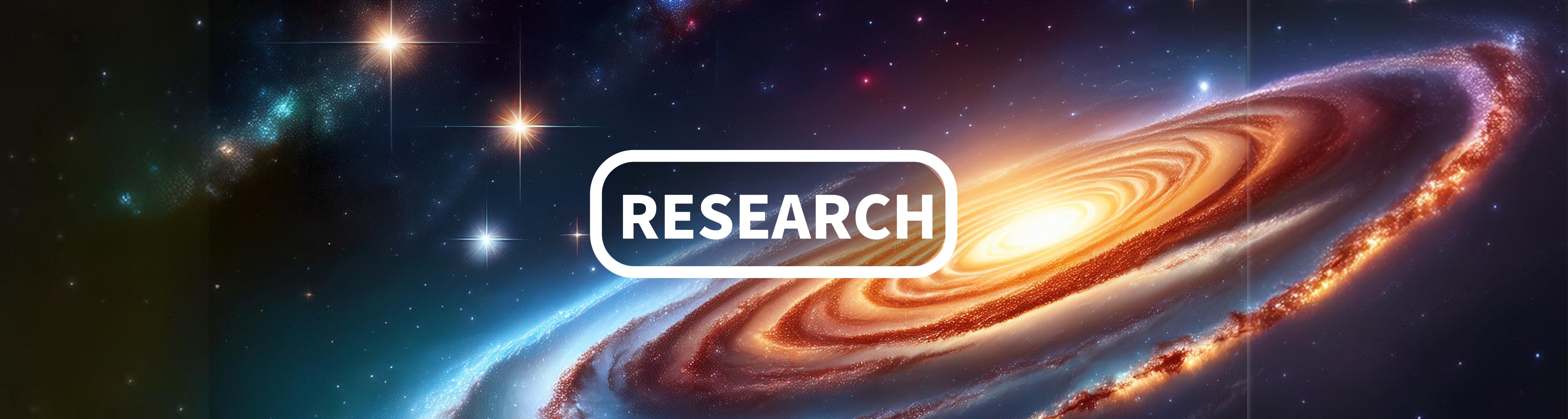
Primary Research Themes
Over the last few years, my research (and that of my mentees) has spanned a few broad themes. Each of these themes is briefly described below. You can click on the buttons corresponding to each theme to explore each topic!
Topics/Buttons with spiny wheels beside them denote work/page-building in progress.
Galaxy Evolution & Cosmology
Novel insights into galaxy evolution and cosmology using large-volume surveys and robust statistics.
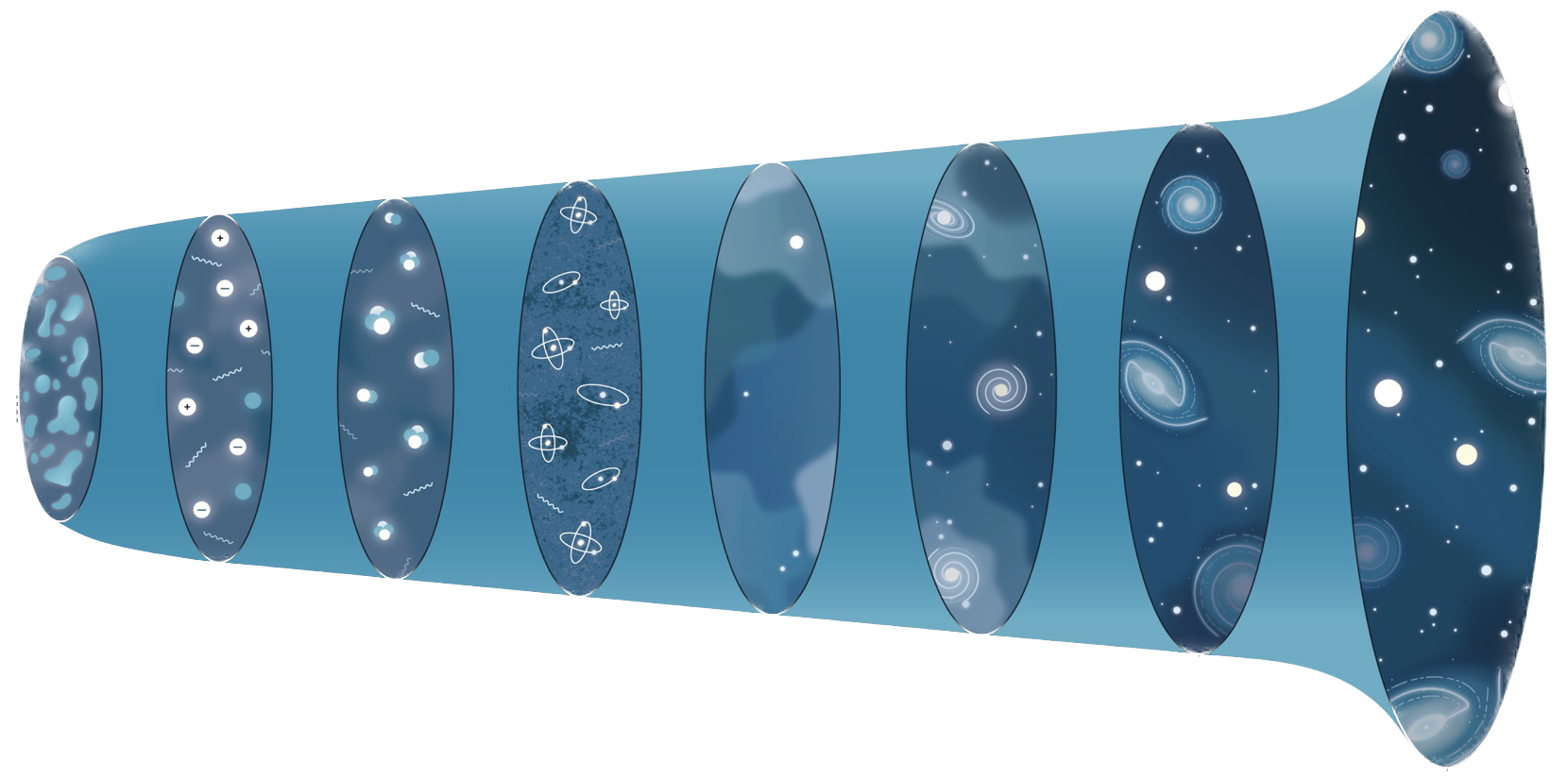
Using Galaxy Structure
The structural parameters of galaxies and their diverse morphological features have played an instrumental role to further our understanding of galaxy formation and evolution. I have focused on leveraging the intricate correlations between the structure of a galaxy and its other attributes (e.g., stellar mass, AGN activity, environmental density) for millions of samples to investigate:- (a) different baryonic processes within galaxies, (b) the effect of dark matter halos on galaxy evolution, and (c) AGN-galaxy co-evolution.
Using Mergers & LSB features
Deep-Wide optical surveys of the sky (e.g., HSC, Rubin-LSST) are pushing the observations of galaxies to lower surface brightness limits than has ever been available over such large volumes. I am currently working on developing roust techniques to detect a wide variety of low-surface brightness features and mergers at various stages. We are using these to gain a better understanding of:- (a) the outskirts of galaxies, (b) the merger history of lower mass galaxies, (c) the role of tidal stripping in groups and clusters, (d) the lowest surface brightness dwarfs and their evolution.
Structural Parameter & Morphology Catalogs
Structural parameters with robust uncertainties for millions of galaxies & AGN hosts
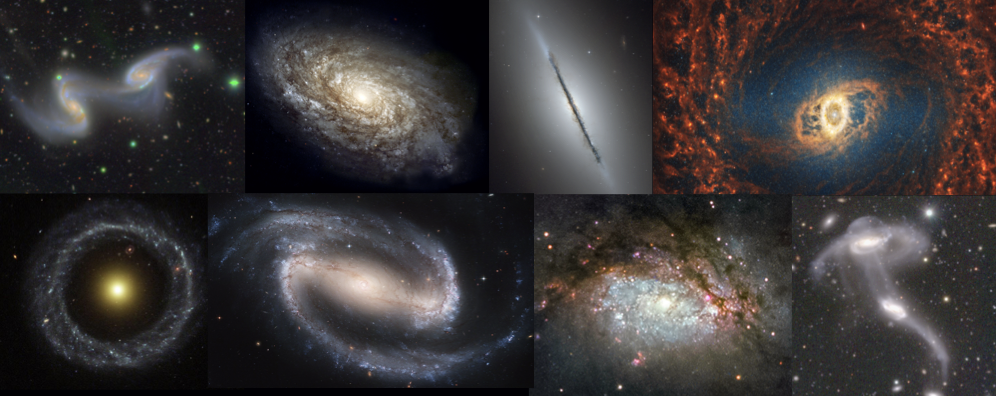
Since the mid-20th century, astronomers have used the structure/morphology of galaxies and AGN hosts to gain a better understanding of how galaxies form and evolve. Thus, very large public catalogs of galaxy structure are essential tools in investigating existing pathways of galaxy evolution and introduce new evolutionary mechanisms.
I have focused on developing novel Bayesian machine learning frameworks to determine the structure of millions of galaxies and AGN hosts. In particular, I have focused on enabling these frameworks to make robust predictions with well-calibrated uncertainties; while necessitating minimal pre-analyzed training data. We have used these frameworks to produce one of the largest structural parameter catalogs till date, containing $\sim 8$ million Hyper Suprime-Cam (HSC) galaxies.
- Structural Parameters for ~8 Million HSC Galaxies
- GaMPEN
- HSC AGN Host Galaxy Morphology
- PSFGAN
- SDSS & CANDELS Morphology Catalog
- GaMorNet
Image-Based Anomaly Detection
Hunting interesting needles in imaging haystacks
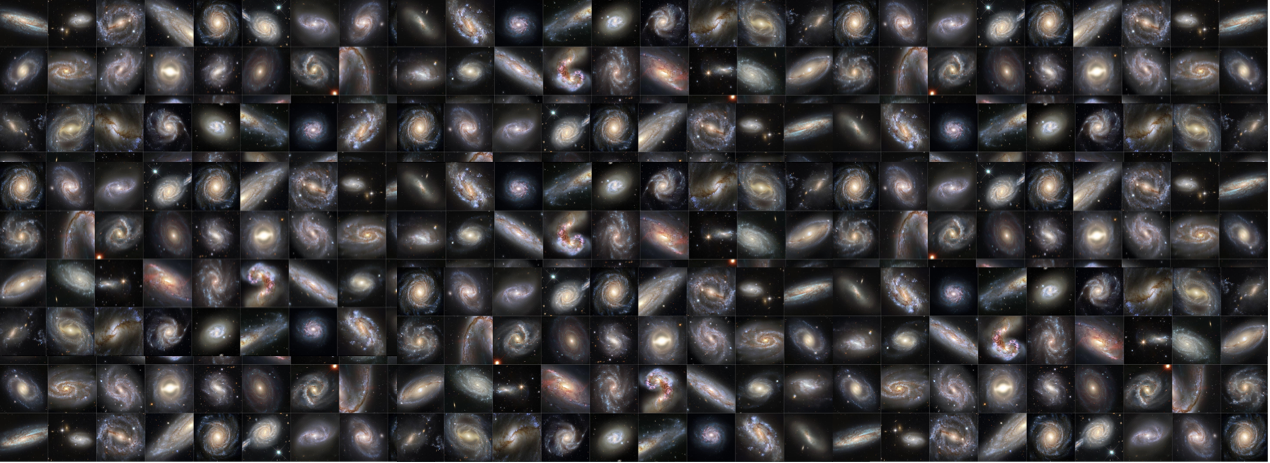
The depth and large area of current/upcoming ground and space-based optical/NIR missions (e.g., Euclid, NGRST, Rubin-LSST) will allow us to find the rarest and most interesting distant objects in the Universe out to a redshift beyond 7 when the Universe was only 5% of the age it is today. Although these missions are uniquely positioned to image these rare objects; finding these small needles in the haystack of TBs imaging data would be impossible without robust algorithms to find rare and interesting objects.
I am currently collaborating with LINCC Frameworks engineers to develop a comprehensive anomaly detection framework for large astronomical imaging datasets.
Robust Machine Learning in Astronomy
Developing & testing bespoke algorithms specifically for astronomy
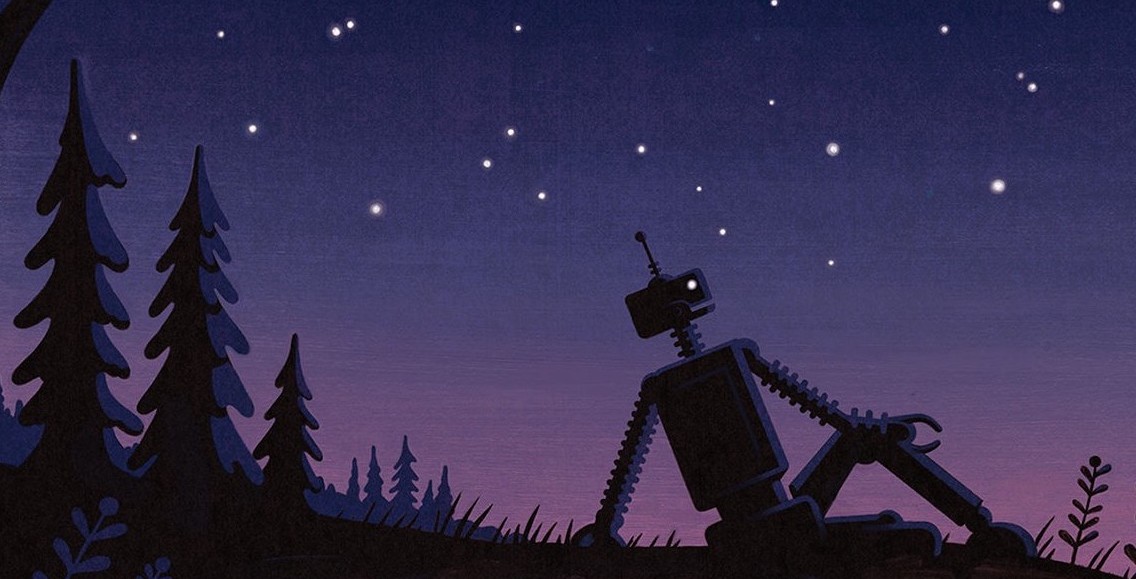
With the emergence of wide-area all-sky surveys, the amount of data in astronomy has been growing at an unprecedented rate. This has led to machine learning (ML) techniques being increasingly employed by astronomers for a wide variety of tasks -- from identifying exoplanets to studying galaxies and black holes.
However, using generic ML tools off-the-shelf as black boxes is dangerous if we do not understand their accuracy/bias/limitationsspecifically in the context of astrophysics. Thus, I have have focused on developing ML frameworks specifically for astronomical applications, enabling these frameworks to estimate robust uncertainties, and stress-testing these frameworks to understand their limitations.